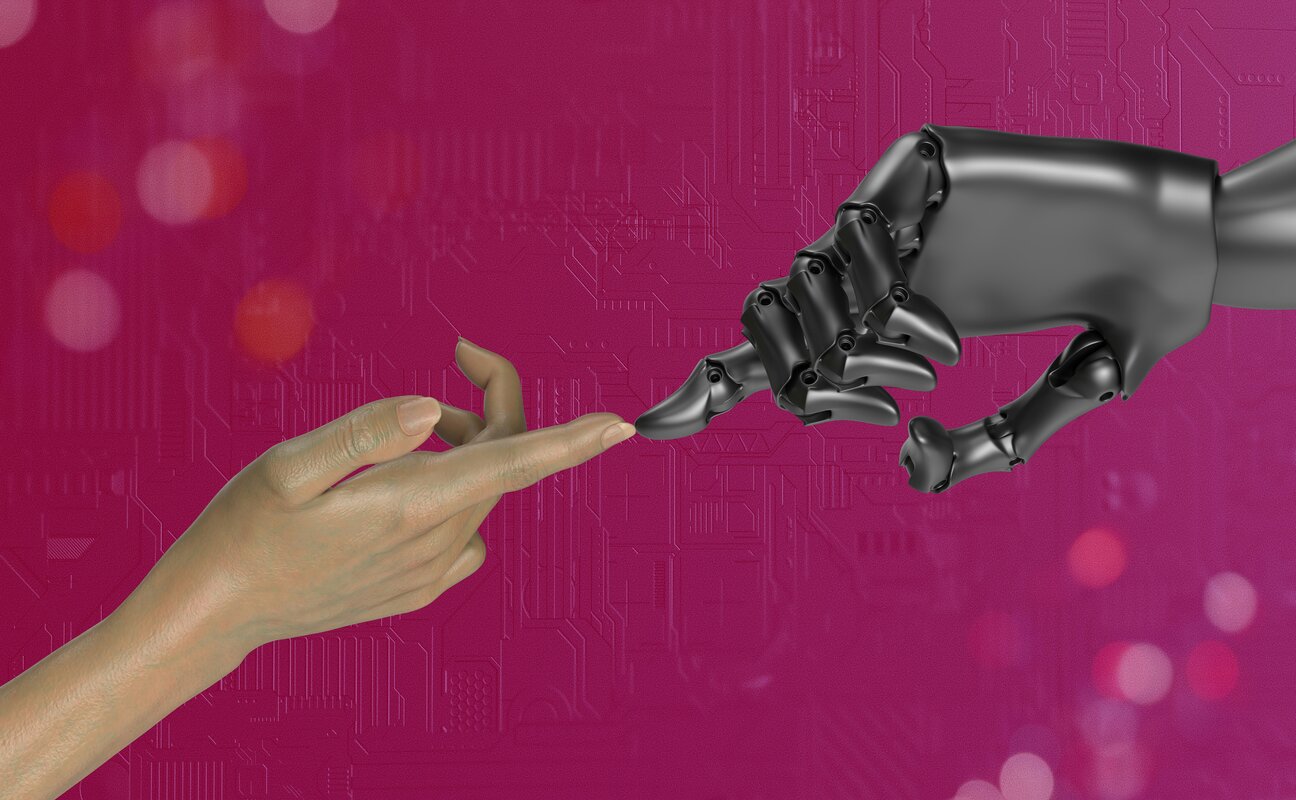
Recently, some of our clients approached us for advice on potential AI offers from both their EMRs and standalone AI solutions for tasks such as Ambien Note taking. Although the integration of Artificial Intelligence (AI) into Electronic Medical Records (EMRs) has evolved significantly in recent years with the promise of enhancing clinical efficiency and patient care, in the smaller practices, the promise has yet to be fulfilled.
According to Gartner’s 2023 Hype Cycle, AI in healthcare is transitioning from the “Peak of Inflated Expectations” to the “Trough of Disillusionment.” While EMR vendors heavily market AI, fewer than 15% of U.S. practices report meaningful clinical AI integration (JAMA Health Forum, 2023).
Should we sign up for the ride now?
Current State of AI in EMRs:
Most of the AI ventures focus on delivering three capabilities: documentation, process automation, and risk stratification.
- Enhanced Documentation: AI-powered medical scribes are now capable of automating clinical notes and medical documentation. Many AI start-ups, such as AvodahMed AI, have developed Ambien AI solutions that automate these tasks, allowing clinicians to focus more on patient care. The technology is already implemented in many healthcare systems, including rural and children’s hospitals across the U.S. Per Reuters, research indicates that AI can generate postoperative reports with greater accuracy than surgeons, reducing documentation errors and enhancing patient safety.
- Operational Efficiency: AI is being utilized to automate routine administrative processes such as scheduling, billing, and data abstraction. This automation allows healthcare professionals to dedicate more time to patient care, thereby improving overall efficiency. The most promising automation is on pre-authorization, which is a main challenge of claim denials. Startups like Cohere Health use LLMs to auto-generate insurance pre-auths, reducing denial rates by 30% (Health Affairs, 2024).
- Predictive Risk Stratification: Tools like Epic’s Deterioration Index use AI to flag at-risk patients, but adoption is low in small practices due to data infrastructure needs.
- Beta Features: EMRs often test AI tools in beta with select clients (e.g., “Athena AI Assistant” for clinical note summarization or prior authorization automation). These may include diagnostic suggestions or marketing insights, but they’re typically gated behind opt-in agreements and NDAs.
While promising, the adoption rate is not as high as it could be. The main barriers are:
- Interoperability: FHIR standards are improving data sharing, but siloed EMR ecosystems (e.g., Epic vs. Cerner) limit cross-platform AI tools.
- FDA Oversight: Only 12 AI/ML tools for EMRs are FDA-approved (e.g., Paige Prostate for pathology), focusing on narrow tasks like imaging analysis. Most documentation/coding tools operate in a regulatory gray area.
- Explainability: Tools like IBM Watson’s “AI FactSheets” aim to demystify decisions, but a 2023 Lancet Digital Health survey found 78% of clinicians distrust AI outputs without transparency.
- Political barriers: Most EMRs are protecting their “turf.”. When it comes to integration, collaboration tasks such as data mapping can be the highest barrier.
For the smaller practices, the threshold for adoption is even higher.
- Implementation Costs: Small practices often face financial constraints that make the adoption of AI technologies challenging. The initial investment for AI-powered EMR systems can be substantial, encompassing costs for technology acquisition, staff training, and ongoing maintenance. Even for smaller AI projects like Ambien Recording, it faces significant ROI uncertainty. A 2024 MGMA report found that AI scribes cost 300–300–800/month per provider but save 2–4 hours/week. Break-even occurs only if freed time is monetized (e.g., seeing 2–3 extra patients daily). In addition, there are hidden costs, e.g., training (~20 hours/provider) and data migration (e.g., 10k–10k–50k for legacy EMRs), which are often overlooked.
- Integration Complexity: Integrating AI into existing EMR systems can be complex and time-consuming. Small practices may lack the necessary IT infrastructure and expertise to seamlessly integrate AI solutions, leading to potential disruptions in workflow during the transition period.
- Data Privacy and Security: The use of AI involves handling sensitive patient information, raising concerns about data privacy and security. Ensuring compliance with regulations such as HIPAA is crucial to protect patient data from breaches and unauthorized access. In the case of Ambien AI, although practices inform the patients that their conversations will be recorded throughout the visit, the consent may be given without the understanding that the full recording might be stored on Cloud for 6 years.
- AI Product Maturity: The AI product design is still in the forming stage. For example, the recommendation of treatment plan might not be AI-generated but “hard-coded” parameters, weights, and induction logic, e.g., certain combinations of symptons as parameters, duration and severity as weights, and diagnosis notes or/and treatment plan as outputs of inductions. Without high-quality inputs from practices, it is highly unlikely that the recommendation algorithm will produce trusted output. Given the small size of the practice, the providers and staff tend to be too busy to be able to commit time to provide inputs for these algorithms.
So what do we recommend for smaller practice clients?
- Selective Adoption: When they are presented with AI capabilities, we suggest that they focus on implementing AI tools that offer immediate and tangible benefits. For example, the Ambien AI/or medical scribe AI promises to reduce the time spent on record-keeping, allowing physicians to allocate more time to patient care. Some of the AI medicalscribes automate up to 75% of documentation tasks, thereby enhancing efficiency. In addition, we noticed improvement in the prompt case closing from the providers compared to before the implementation at some of our clients. Start with Revenue Cycle AI (e.g., billing/coding tools) for immediate ROI.
- Join Pilot Programs: Epic’s Leap program offers discounted AI tools for feedback. For clients with Athena, we suggest that they get together with their success managers to understand their AI Beta offerings. The vendor sessions, in addition to the feature demos, can disclose training data sources and error rates.
_____________________________
Conclusion:
AI integration into EMRs is no longer a distant promise but a present reality with practical applications that can enhance efficiency and patient care. However, in our line of work, we have not yet seen any really meaningful, well-tested, end-to-end AI capabilities in EMR, but the vendors are working on it. Even if they roll out more AI capabilities, do not underestimate the cost of implementation, including potential additional licensing cost, training of staff, review of data hygiene, etc.
In short, at this very moment, it may not be the best timing to engage as things are moving very fast with new models and access cost changing on a weekly basis. For most of our clients who are in the small/medium size category, it might be wiser to observe till the “dust settles down” a bit.
With that said, by staying informed and selectively implementing AI solutions, small practices can join at the right time to take advantage of the technological breakthrough.
If you would like to assess AI opportunites in your practice before making significant investment, contact us either through the website or email us at contact@solutions4billing.com for a 30 minutes FREE consultation session.